The State of Machine Learning
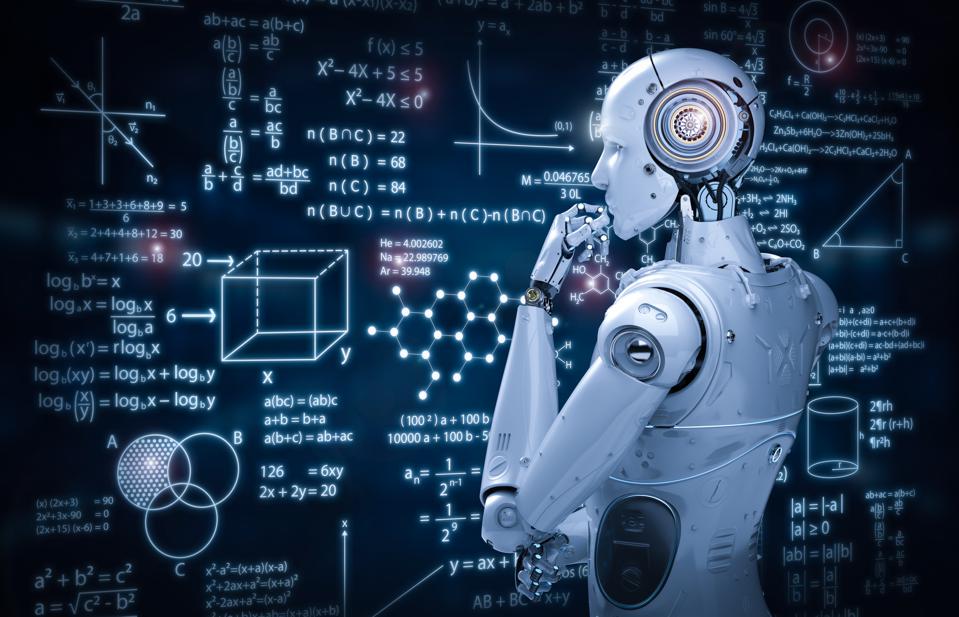
The state of machine learning (ML) is currently at an exciting and rapidly evolving stage. In recent years, advances in technology have led to significant improvements in the capabilities and applications of ML, making it one of the most promising fields in technology today.
One of the key drivers of the recent growth in ML has been the availability of large amounts of data. With the rise of the internet and the growing ubiquity of smartphones, more data is being generated than ever before. This has allowed researchers to train ML models on vast amounts of data, leading to significant improvements in the accuracy and performance of these models.
Another key driver of the growth in ML has been the development of powerful new algorithms. In particular, deep learning algorithms, which are based on neural networks, have become increasingly popular in recent years. These algorithms have been used to achieve breakthroughs in areas such as image and speech recognition, natural language processing, and gaming.
The growth in ML has also been driven by the increasing availability of powerful computing resources. Cloud-based services from companies such as Amazon, Google, and Microsoft now provide developers with access to large amounts of computing power and storage, making it much easier to train and deploy ML models.
The field of ML has also been further advanced by the development of new tools and frameworks, such as TensorFlow, PyTorch, and Keras, which make it easier for developers to implement and experiment with different ML algorithms.
The advancement in ML has led to many new applications and use cases. One of the most significant use cases of ML is in the area of autonomous vehicles. Self-driving cars powered by ML are becoming increasingly common, and are expected to revolutionize the transportation industry. Other areas where ML is being used include healthcare, financial services, and retail.
Despite all the advancements, there are still many challenges in the field of ML. One major challenge is the lack of interpretability of many ML models. Many models are difficult to understand and interpret, making it challenging to understand why they are making certain predictions. Another challenge is the ethical and societal implications of ML, such as issues of privacy and bias.
In conclusion, the state of ML is currently at an exciting stage, with significant advancements in recent years. The growth in ML is being driven by the availability of large amounts of data, the development of powerful new algorithms, and the increasing availability of powerful computing resources. Despite the challenges that still exist, the field of ML has the potential to change the way we live and work for the better, and is expected to be a key driver of innovation in the coming years.